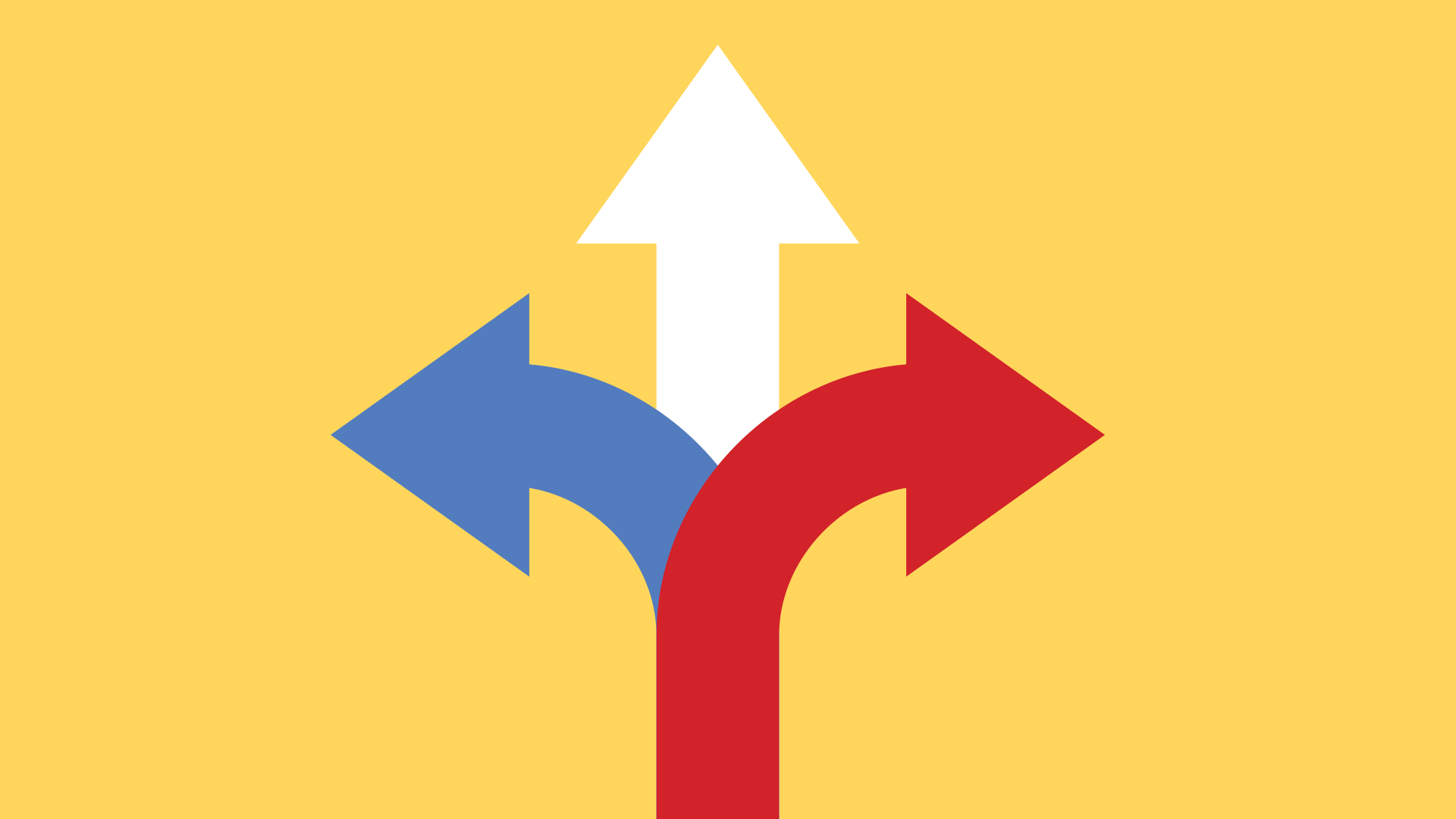
Adaptive Learning 3.0: Finding the Right Learning Pathway for ALL Learners
Fiction is full of characters that break the mold:
Belle left that “poor, provincial town” and Gaston behind to marry the Beast. Bilbo Baggins did a most un-hobbit-like thing and went on an adventure. And Arya Stark would rather water dance than worry about her marriage prospects
The story always gets interesting when our heroes don’t follow the path that’s set for them.
The same is true in learning.
When employees have the opportunity to make choices over their learning things get interesting. In fact, numerous studies show that giving employees more control over their own learning results in a workforce that’s more motivated, more engaged, more loyal and even higher performing. (You can read more about choice here and here.)
Adaptive Architecture: Pre-Set Pathways
But even in the most advanced learning systems, “choice” is hard to come by. For example, many of today’s adaptive learning technologies insist that users follow one of the following formulaic pathways:
Content First– Within this pathway, all users must review the content prior to getting to the learning practice or assessments, regardless of their prior knowledge or experience. This approach is fine for learners who have little prior knowledge, because it allows them to fully review the content before diving into an assessment. But for learners who already know the material, this approach can result in a very negative learning experience. By forcing employees to repeat information they already know, they can quickly grow frustrated, disengaged and bored – which can harm their future performance, slow down their learning experience and cause them to view the training (and future training) as “a waste of time.”
Questions First– Within this pathway, all users must answer a series of questions before they can access the corresponding content. Learners who have prior knowledge will be able to move through the assessment questions and demonstrate mastery quickly. But for learners who have little prior knowledge or experience, the road might not be nearly as smooth. These learners will likely struggle with questions they simply don’t know the answers to (because they haven’t reviewed the content yet), causing them to feel discouraged, anxious and less confident.
Also, while some learners want to see the questions first, others prefer to dive right into studying the content. In fact, we’ve found that the breakdown is pretty even between the entrance pathways learners choose within our platform:
These architectures force employees to enter the learning program through a particular pathway way, regardless of where they fall within the knowledge spectrum. This results in a less-than-optimal and less autonomous user experience. It would be as if Belle’s father forced her to marry Gaston. Or as if Gandalf never even asked Bilbo if he wanted to join the dwarves on their quest.
So why do adaptive programs use this pre-set architecture?
Lots of adaptive systems rely on these pre-set pathways because they’re necessary to inform the branching or algorithms that the systems use to adapt the content. For example, an adaptive system based on advanced algorithms might use a learner’s answers to an initial set of questions to determine what content needs to be presented next.
But we’re entering a new era of adaptive systems – what we call adaptive 3.0. In this new era, adaptive technologies are leveraging AI and machine learning to make complex adaptations in real time. This means that new adaptive systems have much more flexibility in how they design their entrance architecture. As systems move away from adaptivity that’s based on branching or advanced algorithms toward more AI-enabled pathways, they’ll no longer have to insist that learners answer a series of questions first or review the content before they can pass out of it. They’ll also be free to support more opportunities for employees to control aspects of their learning.
Adaptive Choice in Action
For example, our adaptive platform leverages cutting-edge AI and machine learning capabilities to inform its adaptations. Our system doesn’t require a base set of data (e.g. from an initial series of questions) to design learning pathways. Rather it uses real-time analytics to determine the depth and degree of the material that’s appropriate for each learner.
As a result, within our system, learners are free to choose how they want to interact with the learning from the get-go. As soon as learners enter a course, they have the choice to, for example, read the content, watch it, go straight into practice assessments or do a combination of all three. They can design their own learning pathways based on their experience, knowledge, time constraints and learning preferences.
If a learner has prior experience with the topic, they can move straight into the practice assessments. They don’t have to waste time cycling through content they already know. Conversely, if a learner has little prior knowledge, they can spend their time reviewing the learning within the reading or watching modalities before they move on to the assessment portion.
And here is where the story gets really interesting. By allowing learners to choose how they want to engage with the content and chart their own learning path, our system delivers dramatic learning efficiencies like:
- A 55% reduction in training times
- 38% knowledge improvements
- Nearly 60% fewer on-the-job errors
- 81% positive user feedback (in what was previously considered “boring old compliance training” no less)
Breaking the mold and setting one’s own path – whether it be in fiction or in learning – requires a good support system. Belle had her father, Bilbo had Gandalf, Ayra had Ned…and learners have Fulcrum. For more information about how Fulcrum Labs leverages AI and machine learning within its platform to deliver more effective learning and more opportunities for learner control, let’s connect and set up a demo.
You might also be interested in:
Adaptive 3.0: Using AI to rework Confidence in Adaptive Learning